By James Ballard
Date Published: April 17th, 2025
15 minutes
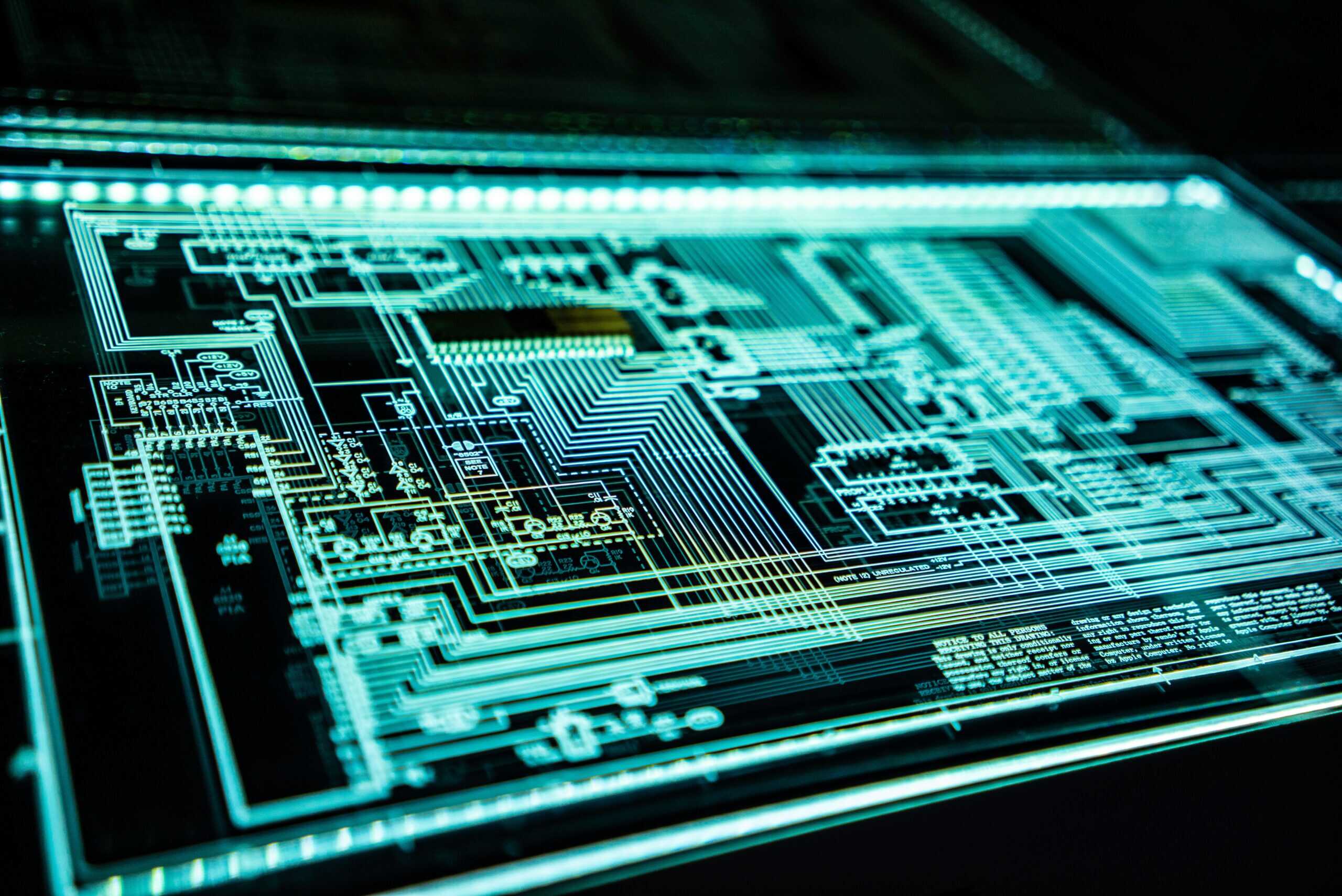
Introduction: AI in Cybersecurity: How Machine Learning Is Transforming Threat Detection
In an era where cyber threats are growing in frequency and complexity, artificial intelligence (AI) has emerged as a game-changer in the field of cybersecurity. Machine learning algorithms, in particular, are revolutionizing how Security Operations Centers (SOC) and incident response teams detect and respond to advanced threats, including ransomware, phishing, advanced persistent threats (APTs), and zero-day vulnerabilities. By harnessing predictive analytics, behavioral analysis, and real-time threat intelligence, AI-powered cybersecurity solutions can mitigate risks faster, reduce human error, and offer proactive defense strategies against evolving cyberattacks.
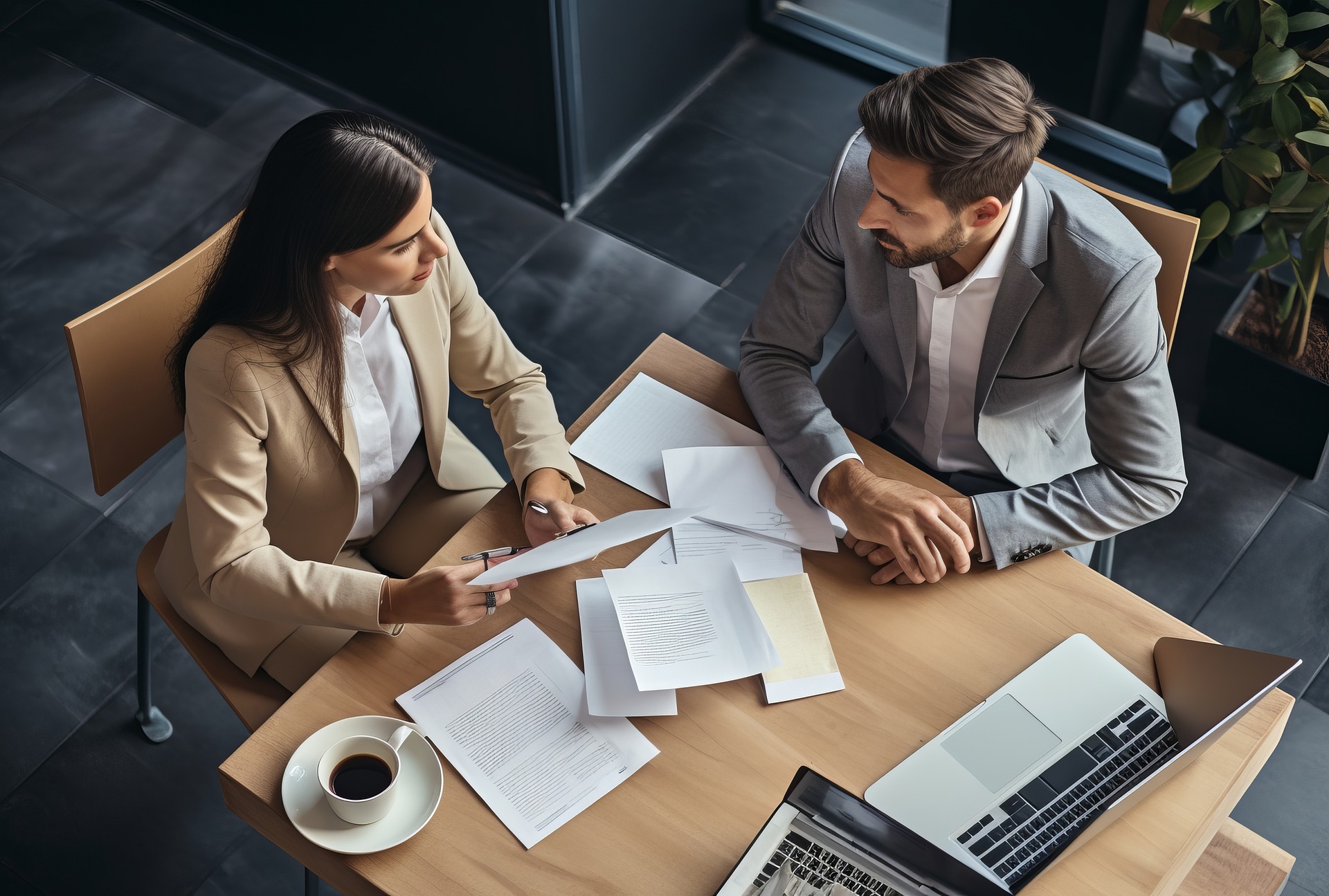
1. The Growing Importance of AI in Cybersecurity
AI and the Evolving Threat Landscape
The cyber threat landscape is becoming more diverse and sophisticated due to factors like cloud computing adoption, the rise of IoT (Internet of Things), and the expansion of remote work. Traditional antivirus solutions and manual intrusion detection systems often struggle to keep pace. AI in cybersecurity bridges these gaps by leveraging machine learning models that continuously learn from real-world data, identifying anomalies and attack patterns that might slip past conventional filters.
Why Machine Learning Is the Future
- Scalability: As organizations accumulate massive datasets—such as network traffic logs and system event data—machine learning ensures that analysis scales without a proportional increase in staff or time.
- Accuracy: AI-driven threat detection leverages deep learning and natural language processing (NLP) to identify malicious behaviors with higher accuracy than rule-based systems.
- Adaptive Defense: Machine learning evolves with new attack vectors, adapting to patterns that were previously unseen. This continuous improvement helps maintain cyber resilience even as cybercriminals refine their tactics.
2. Key Machine Learning Applications in Threat Detection
Deepfake technology is being used not just for misinformation but also for fraudulent financial transactions and identity theft. Attackers can generate highly realistic video and voice clones to manipulate employees, executives, and even biometric authentication systems.
Behavioral Analysis for Insider Threats
Insider threats can be hard to detect with static rules alone. Behavioral analytics powered by machine learning establishes a baseline of user behavior, such as login times, resource access patterns, and data transfer volumes. Any anomalous activity, like accessing a sensitive database at unusual hours, triggers a warning. This real-time monitoring drastically reduces the time it takes to detect a potential breach.
Predictive Analytics for Advanced Persistent Threats (APTs)
Advanced persistent threats are stealthy attacks, often orchestrated by state-sponsored groups, that lurk in networks for extended periods. Predictive analytics can identify the subtle indicators of APT activities, such as slight deviations in network traffic or irregular process execution. By applying machine learning algorithms to these data points, cybersecurity teams gain an early warning system for sophisticated intrusions.
Threat Intelligence and Automated Response
Modern Security Information and Event Management (SIEM) platforms integrate AI-driven threat intelligence feeds to automatically correlate security alerts from multiple sources. When malicious behavior is detected, AI-based solutions can execute automated playbooks—blocking suspicious IP addresses, isolating infected endpoints, or quarantining compromised virtual machines—all without waiting for human intervention. This automated response capability is critical for containing ransomware attacks before they spread.
3. Benefits of AI-Driven Cybersecurity Strategies
- Early Threat Detection: Machine learning models excel at spotting irregularities in network traffic, user activities, or system logs—often catching cyber threats in their earliest stages.
- Reduced False Positives: AI can distinguish between legitimate user behavior and actual malicious activity, alleviating alert fatigue and helping security professionals focus on real threats.
- Proactive Defense: Predictive algorithms anticipate future attack patterns, enabling preemptive mitigation rather than reactive clean-up.
- Enhanced Compliance: Automated compliance monitoring ensures adherence to regulations like GDPR, CCPA, and HIPAA by flagging unusual data handling or unauthorized access to sensitive information.
- Resource Efficiency: AI lowers the burden on human analysts, allowing SOC teams to allocate resources more effectively and handle higher volumes of security events.
4. Challenges and Considerations in AI Cybersecurity
While AI in cybersecurity offers transformative benefits, organizations must navigate key challenges:
- Data Quality: Effective machine learning depends on comprehensive, high-quality datasets. Poor or incomplete data can lead to false negatives or false positives.
- Model Explainability: Complex deep learning models can be black boxes. Some regulatory environments require transparent decision-making processes, so choosing interpretable models or employing XAI (Explainable AI) techniques is increasingly important.
- Adversarial Attacks: Cybercriminals are exploring ways to trick machine learning systems, such as adversarial examples designed to evade detection. Regular model updates and robust defensive training can mitigate this risk.
- Integration Complexity: Incorporating AI-driven solutions into legacy infrastructure may require significant IT investments, staff training, and updates to incident response protocols.
5. The Future of AI-Powered Cybersecurity
AI in cybersecurity is set to become even more advanced, with emerging fields like federated learning improving data privacy, reinforcement learning enhancing automated threat responses, and quantum computing eventually altering encryption standards. Organizations that invest early in machine learning-based threat detection will be better prepared for the next wave of cyber threats, maintaining cyber resilience in an ever-changing digital landscape.
Start working with our cybersecurity experts.
Conclusion: Embrace AI for Proactive Threat Detection
In a world where cyberattacks grow more sophisticated daily, machine learning represents a powerful ally in proactive threat detection and risk mitigation. By integrating AI-driven security tools—ranging from behavioral analytics and predictive intelligence to automated incident response—organizations can shorten detection times, reduce operational costs, and maintain tighter security perimeters. Adopting AI in cybersecurity is no longer optional; it is a strategic imperative for any business seeking to safeguard its assets, reputation, and customer trust.
Key Takeaways:
- AI enables fast, accurate threat detection by analyzing vast datasets in real time.
- Machine learning reduces false positives and alert fatigue, allowing security analysts to focus on critical tasks.
- Predictive analytics identifies hidden attack patterns, giving organizations a head start against advanced threats.
- Automated responses contain attacks before they proliferate, minimizing damage and maintaining operational continuity.
- Future innovations in AI and quantum computing will further enhance cyber defenses, ensuring a more secure digital ecosystem.
Need help securing your organization? Contact Cryptek today for a free security assessment and consultation.